NSF Supports Machine Learning in Music Information Retrieval
July 5, 2022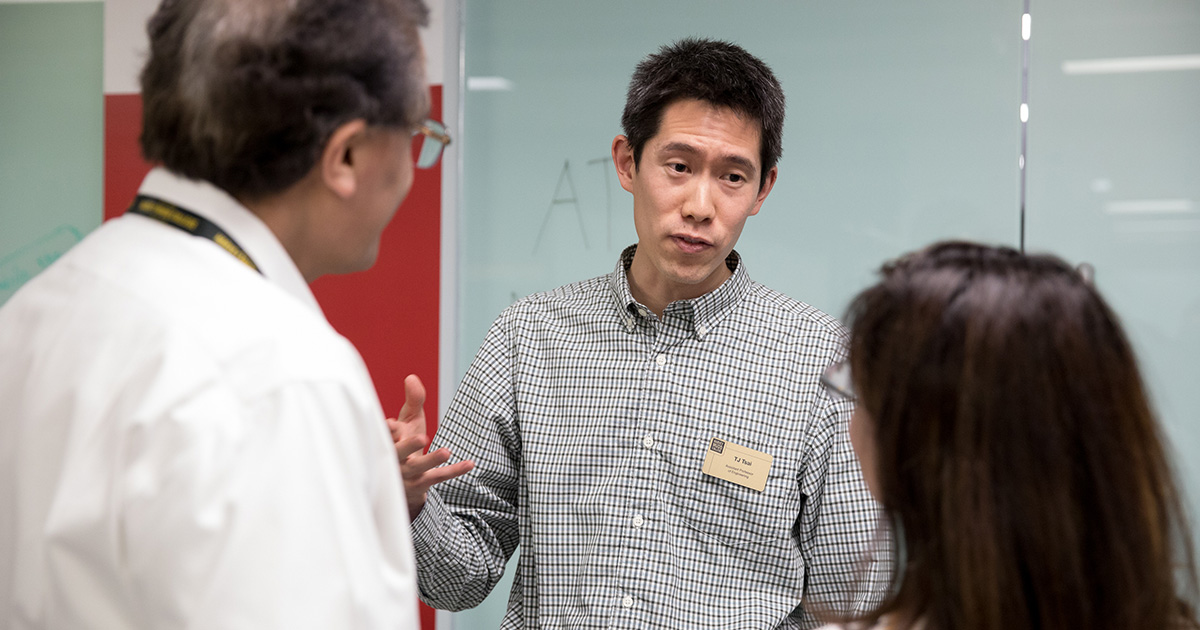
The field of music information retrieval sits at the intersection of music, signal processing and machine learning. “We use interesting problems in music as a playground to develop mastery of tools and techniques in signal processing and machine learning,” says Harvey Mudd College engineering professor TJ Tsai, who received a $500,270 CAREER award from the National Science Foundation for his project “Ordered Alignment Methods for Complex, High-Dimensional Data.”
“Some examples of problems we have investigated in the past include teaching a machine to follow along in sheet music as it listens to an audio performance, predicting the composer of a piece of music based on its compositional style and taking a cell phone picture of a page of sheet music and being able to hear what it sounds like,” Tsai says.
The focus of Tsai’s proposal is to design ordered alignment methods that are scalable enough to be used in interactive music applications, are flexible enough to handle complex, structured music data, and can be integrated into the training process of modern neural networks.
“Modern machine learning models often require a lot of training data, and a lot of data nowadays is sequential data like text, audio and video,” Tsai says. “To train models, we often need to know the correspondence (or ‘alignment’) between two sequences of data. For example, given an audio recording of a person speaking and a text transcription of what they say, we would like to know when in the audio recording the person is saying a particular word. Or, given a video and a sequence of actions/events that occur in the video, we would like to know when a particular action/event occurs in the video. This type of information is very time-consuming to annotate by hand, and we would like to develop automated tools to do this in a robust, efficient and practical way. This grant explores ways to perform this type of alignment more effectively.”
Music genres that have not been explored very much in his field, like gospel and hip-hop, will be foundational to Tsai’s research. “I would love to partner with students who have domain expertise in these areas, whether it’s dancing in a hip-hop group or singing in a gospel choir,” he says. “In this way, I think this project can take advantage of the diversity in our Mudd community.”
Tsai says that diversity of background and experience is one of the things he appreciates most about the field of music information retrieval. “Music is wonderfully diverse, so anyone from any culture or background can find something that relates to their culture, their musical talents or perhaps just the music that they love to listen to.”
The Faculty Early Career Development (CAREER) Program supports early career faculty who have the potential to serve as academic role models in research and education and to lead advances in the mission of their department or organization. Past HMC faculty recipients include biology professor Danae Schulz (2021), computer science professor Yi-Chieh (Jessica) Wu (2018), computer science professor Jim Boerkoel (2017), physics professor Jason Gallicchio (2020), chemistry professor Lelia Hawkins (2015) and engineering professors Nancy Lape (2009) and Albert Dato (2020).
NSF grants are the largest share of external support for faculty research at Harvey Mudd.